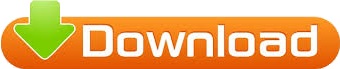
We trained and blindly tested this virtual-staining network using unlabelled kidney tissue sections to generate micro-structured combinations of haematoxylin and eosin (H&E), Jones’ silver stain, and Masson’s trichrome stain. This digital staining matrix is also used to virtually blend existing stains, digitally synthesizing new histological stains. This approach uses a single deep neural network that receives two different sources of information as its input: (1) autofluorescence images of the label-free tissue sample and (2) a “digital staining matrix”, which represents the desired microscopic map of the different stains to be virtually generated in the same tissue section. Here, we present a new deep-learning-based framework that generates virtually stained images using label-free tissue images, in which different stains are merged following a micro-structure map more » defined by the user. Recently, the ability to virtually stain unlabelled tissue sections, entirely avoiding the histochemical staining step, has been demonstrated using tissue-stain-specific deep neural networks.
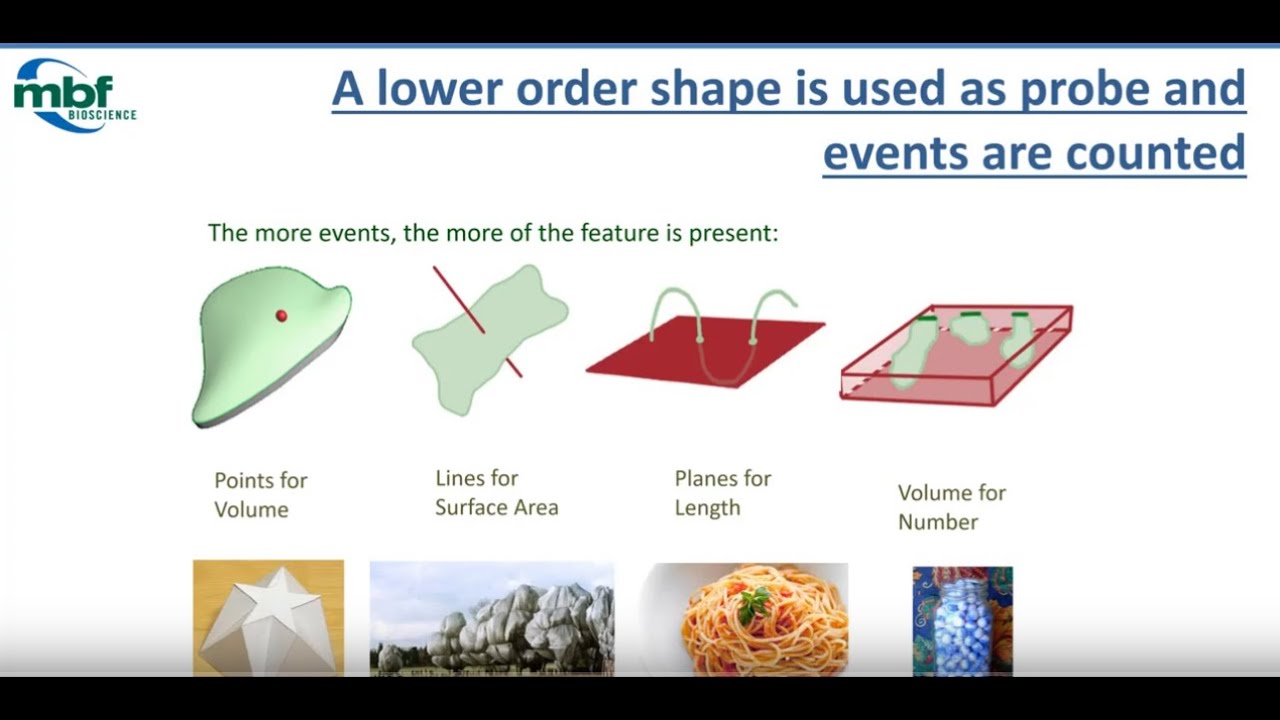
However, this process is time consuming, labour intensive, expensive and destructive to the specimen. Histological staining is a vital step in diagnosing various diseases and has been used for more than a century to provide contrast in tissue sections, rendering the tissue constituents visible for microscopic analysis by medical experts.
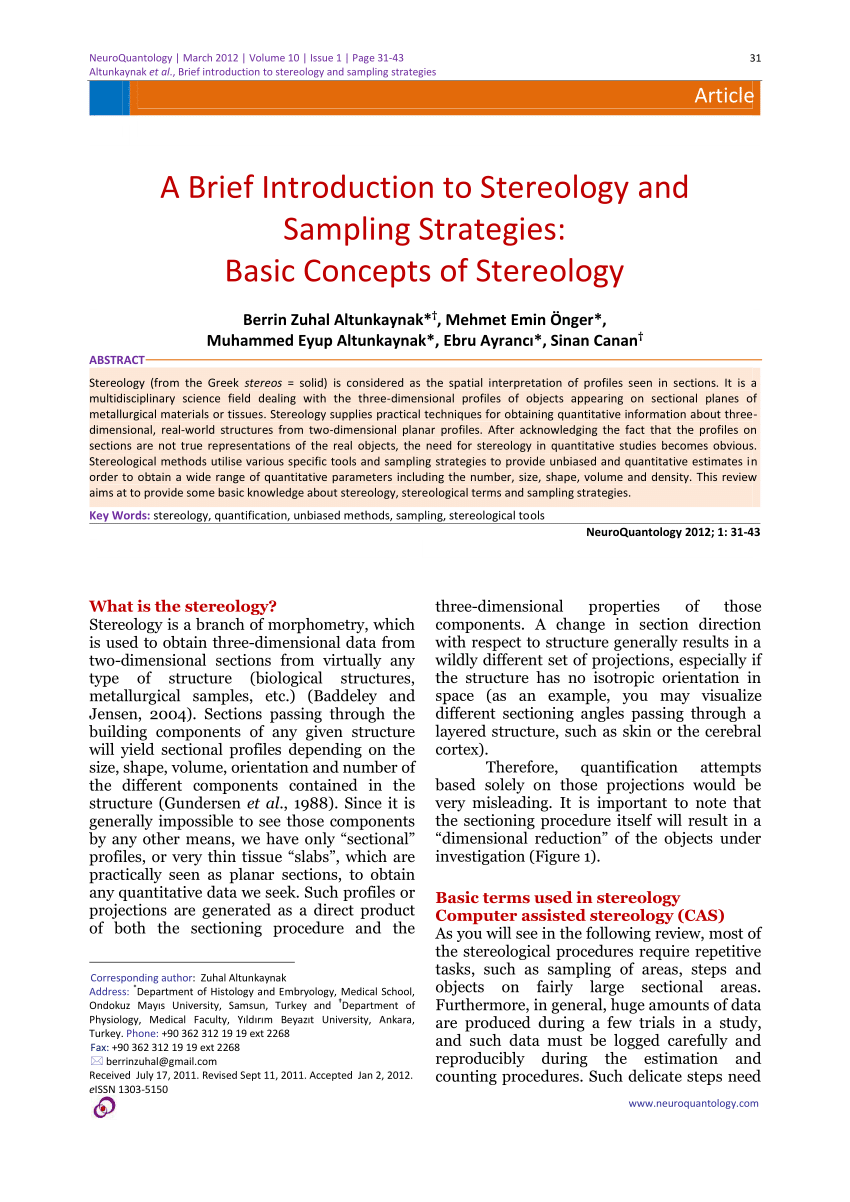
This stain-to-stain transformation framework can improve preliminary diagnoses when additional special stains are needed, also providing significant savings in time and cost. A second study found that the quality of the computationally generated special stains was statistically equivalent to those which were histochemically stained. Based on the evaluation by three renal pathologists, followed by adjudication by a fourth pathologist, we show that the generation of virtual special stains from existing H&E images improves the diagnosis of several non-neoplastic kidney diseases, sampled from 58 unique subjects (P = 0.0095). Here, we demonstrate the utility of supervised learning-based computational stain transformation from H&E to special stains (Masson’s Trichrome, periodic acid-Schiff and Jones silver stain) using kidney needle core biopsy tissue sections. While the hematoxylin and eosin (H&E) stain is most commonly used, special stains can provide additional contrast to different tissue components.
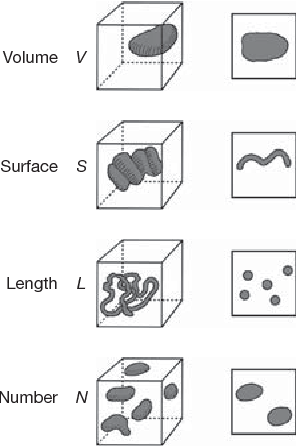

Pathology is practiced by visual inspection of histochemically stained tissue slides.
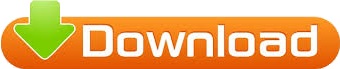